Computational Structural Glycobiology Studies
Glycans are one of the four fundamental classes of macromolecules that comprise living systems, along with nucleic acids, proteins, and lipids.
Glycans are directly involved in the pathophysiology of every major disease such as diabetes, cancer, arthritis, asthma, heart disease, autoimmune disease and infectious disease. Additional knowledge
of glycobiology in the context of the three dimensional structures is needed to the fundamental understanding of biology and pharmaceutical development. However, there is critical knowledge
gap between the importance of glycans and the understanding of many glycan-related biological processes due to the limitations of experimental characterization.
To make progress in this field, I have accomplished a series of computational studies to investigate the molecular mechanism of N-glycosylation, the effects on N-glycans on protein structure and function, and N-glycan conformational space in the Protein Data Bank, and structural diversity and characteristic of glycan binding sites. I have also developed novel computational tools for glycan sequence analysis, glycan structure alignment and similarity measurement, and glycan ligand binding site prediction.
As subsequent research, I am carrying out diverse computational structural glycobiology studies.
Molecular Recognition Study at the Atomic Level
Accurate characterization of ligand binding affinity is indispensable for designing molecules with optimized binding sensitivity and specificity to a target receptor for drug discovery.
Theoretical and computational tools, if they were able to predict quantitative correlations between protein-ligand structure and their binding affinities,
would have a big impact on drug design. However, accurate prediction of ligand binding affinity is still challenging mainly due to protein’s structural flexibility and inaccuracy
of scoring functions.
My approach to make progress in this field is to use atomistic molecular dynamics simulations for calculations of absolute ligand binding free energies. I use alchemical free energy calculations, particularly, free energy perturbation molecular dynamics (FEP/MD) simulations, which use a step-by-step decomposition of the total reversible work. I am especially interested in developing computationally efficient FEP/MD methods and expanding their applicability in practical drug discovery.
Atomistic molecular dynamics simulation is also a powerful technique to understand the underlying basis of molecular recognition by characterizing dynamic and energetic properties during the event. I have been harnessing this technology to elucidate the molecular mechanisms of interactions between biomolecules.
Molecular Recognition Study at the High Throughput Screening Level
Efficient incorporation for receptor flexibility in molecular docking has been the most important issue in virtual high throughput screening (VHTS). In protein-ligand interactions, receptor
flexibility is crucial because the flexible nature of proteins is a key determinant of binding affinity and target specificity of drug activity. However, accounting for receptor flexibility
upon docking calculations is computationally challenging, so in most cases, docking methods treat receptor as completely rigid, having a single fixed conformation. Addressing this major issue,
I have developed a novel docking method for VHTS called “distributed docking” by combining ligand based shape-matching method with receptor based multiple-receptor conformation docking. Benchmark
tests clearly show noticeable improvements in performance while maintaining the computation speed of single-receptor conformation docking.
Another important issue in VHTS is to use predicted protein models in virtual screening. Even though there are a large number of experimentally solved protein structures, a gap still exists in sequence and structure information that prevents researchers from identifying more drug targets. Currently, there has been outstanding progress in the fields of protein structure prediction. Nonetheless, applying the theoretically predicted protein structures to docking experiments is challenging due to structural errors presented near the binding pocket in the model structure, which result in significant drop-off in the ability to recognize ligand in the binding pocket. To tackle this problem, I probed into the development of a new docking method. I have developed a docking method, named “SLIM”. This method measures shape and chemical feature similarity between the inner shape of binding pocket and library compounds in order to predict the binding affinity of the compounds to a target protein. An in-depth analysis showed that the SLIM method not only is extremely faster than conventional docking methods, but also outperforms them against conformational variations of receptors. In particular, when the SLIM method is evaluated against homology-modeled receptor conformations from structure templates with > ~30% sequence identity to the target, it offered significant better enrichment than conventional docking methods.
In a subsequent study, I have developed a blind docking method called “BSP-SLIM”. Specifically, BSP-SLIM acts as an integrated tool in which algorithms for the template-based ligand binding site
prediction are incorporated with SLIM. The method can be applied without any prior information of ligand binding site and is tolerant to structural errors that exist in modeled protein structures.
To prepare a benchmark set of proteins whose homologous protein templates are not available, protein 3D models were generated from the sequences, using threading-based I-TASSER technologies.
Benchmark results evidenced the BSP-SLIM method to produce reliable performance in predicting native ligand poses and prioritizing active compounds against the modeled protein structures.
Molecular Recognition Study at the Proteomic Level
With a rapid increase in the number of high-resolution protein-ligand structures, making the best use of known protein-ligand structures is very useful way to obtain
structural insight into protein-ligand binding. On the basis of the fact that the structurally similar binding sites share information about their ligands, I have
developed a computational tool, G-LoSA. G-LoSA rapidly identifies the largest conserved local structure from a pair of structures regardless of sequence continuity
and protein fold, and then superposes the structures using equivalent residue pairs in the identified local structure. G-LoSA also provides a measurement of structural
similarity between the structure pair.
As a subsequent research, I have developed a template-based ligand binding site (BS) prediction method using G-LoSA. Accurate determination of potential ligand BS is a key step for protein function characterization and structure-based drug design. A large benchmark set validation shows that my method successfully predicts drug-like ligands’ positions and is particularly efficient for accurate detection of local structures conserved across proteins with different global topologies. Recognizing the performance complementarity of the G-LoSA-based method to a template-based ligand BS prediction method using global structure alignment and a non-template geometry-based method, I have developed a robust consensus scoring method, CMCS-BSP, which efficiently integrates computational tools using different BS prediction algorithms. CMCS-BSP shows significant improvement on prediction accuracy.
Once a binding site is determined in a given target protein, the next step is to design molecules that can bind in the binding pocket with high affinity. As another application, I have searched the known BS structure library using G-LoSA to identify ligand BS structures with similar geometry and physicochemical properties to a target binding-site structure. Then the ligands in the identified complexes are used as structure templates to predict/design a ligand for the target protein. The benchmark results clearly showed that using the currently available protein-ligand structure library, G-LoSA is able to identify a single template ligand that is highly similar to the target ligand in more than half of the benchmark targets. In addition, an assembly of structural fragments from multiple template ligands with partial similarity to the target ligand can be used to design novel ligand structures specific to the target protein.
In order to extend its applicability to broad local structure-centric biological studies, G-LoSA has been improved to generate more number of initial alignments by adopting iterative maximum clique search and fragment superposition and to provide length-independent and physicochemical property-based scoring function (GA-score) to measure structural similarity. Our benchmark evaluation shows that the updated G-LoSA outperforms its previous version
My study opens up the potential use of alignment and similarity measurement between local structures in biomolecules for structural bioinformatics study and rational drug design. In particular, G-LoSA enables to accomplish high throughput analyses on local structure conservation across proteins and thus could be a promising computational tool to solve local structure-centric biological problems on a proteomic scale such as polypharmacology and protein-protein interactions network.
Subsequently, I am working on research projects to develop high-performance computational toolset for structure-based protein-ligand interaction studies and drug discovery at the proteomic level.
Design of Novel Therapeutic Agents Using Computational Approaches
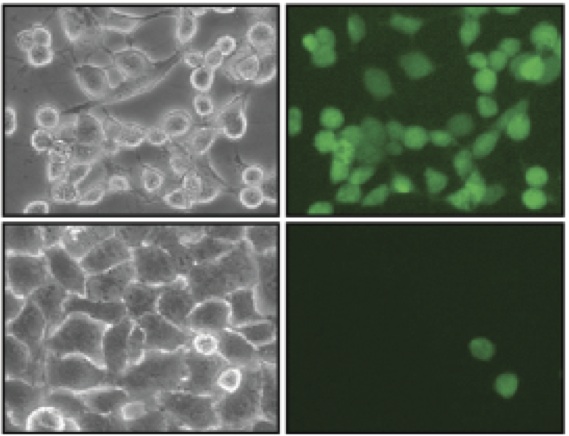
Type 2 diabetes
Besides developing efficient computational methods, their practical applications to drug discover projects are also my major research interest. I conducted researches to discover novel compounds that modulate the functions of PPARγ. It has been known that PPARγ plays an important role in type 2 diabetes, cellular differentiation, atherosclerosis, and cancer. Thiazolidinedione-type treatments are mainly used for patients with type 2 diabetes. However, the drug is not effective in reducing cardiovascular risks that is usually associated with type 2 diabetes and can lead to side effects such as weight gain, fluid retention, edema, etc. As such, researchers have been trying to develop new drug candidates capable of treating multiple related symptoms at once with fewer side effects. Focusing my effort in addressing this issue, I performed computational experiments to discover novel compounds showing biological activity to PPARγ. Consequently, I was able to identify a series of chemical agents that can fully or partially activate PPARγ: (β-carboxyethyl)-rodanine derivatives and 1,3-diphenyl-1H-pyrazole derivatives.
Neurodegenerative disorders
E2–25K/Hip2 is an unusual ubiquitin-conjugating enzyme that interacts with the frameshift mutant of ubiquitin B (UBB+1) and has been identified as a crucial factor regulating amyloid-neurotoxicity. Our collaborators found that disrupting UBB+1 binding markedly diminishes synthesis of neurotoxic UBB+1-anchored polyubiquitin, suggesting that the interaction between E2–25K and UBB+1 is critical for the synthesis and accumulation of UBB+1-anchored polyubiquitin, which results in proteasomal inhibition and neuronal cell death. For the development of new approaches to the treatment of Alzheimer disease, Huntington disease, and other neurodegenerative disorders, I carried out computational studies to design novel compounds that can inhibit E2-25K/Hip2 and UBB+1 interactions. Through a series of computational experiments, which consist of ligand binding site prediction, SLIM-docking, MD simulation, multiple receptor conformation docking and ligand-based structure search, I was able to successfully identify an active compound with 25 nM IC50.
Cancers
α-Helices play a critical role in medicating many protein-protein interactions (PPIs) as recognition motifs.
There has been of great interest in developing non-peptidic small molecules that can mimic short α-helical peptides to modulate such PPIs.
In an effort to discover novel drug-like molecules inhibiting PPIs of MDMX and MCL-1, which are drug targets for cancer treatment,
I have carried out computational analyses on the experimental results and virtual high-throughput screening for computationally generated α-helix mimetic-focused compound
library.
I am also working on computer aided drug discovery, aiming to design small molecules for modulating the functions of protein phosphatases and WAVE regulatory complex.
Infectious diseases by Viruses
RNAi has been examined as a novel technique for the discovery of efficient antiviral agents. A major problem in antiviral therapy using siRNAs targeting RNA viral genome, however, is high sequence diversity and mutation rate due to genetic instability of the viral genome. To overcome this problem, I developed a novel program, called “CAPSID” (Convenient Application Program for siRNA Design), to design siRNAs targeting highly conserved regions within RNA viral genomes. CAPSID was applied to design siRNAs simultaneously targeting multiple strains of Human enterovirus B (HEB), which is connected to aseptic meningitis, encephalitis, cardiomyopathy, and diabetes. The siRNA developed during the study had high antiviral efficacy that could decrease virus replication and hinder cytotoxicity in cells that various reference strains and clinical isolates of HEB were treated. A cell resistance to emerging viable escape mutants and sustained antiviral ability were also observed.